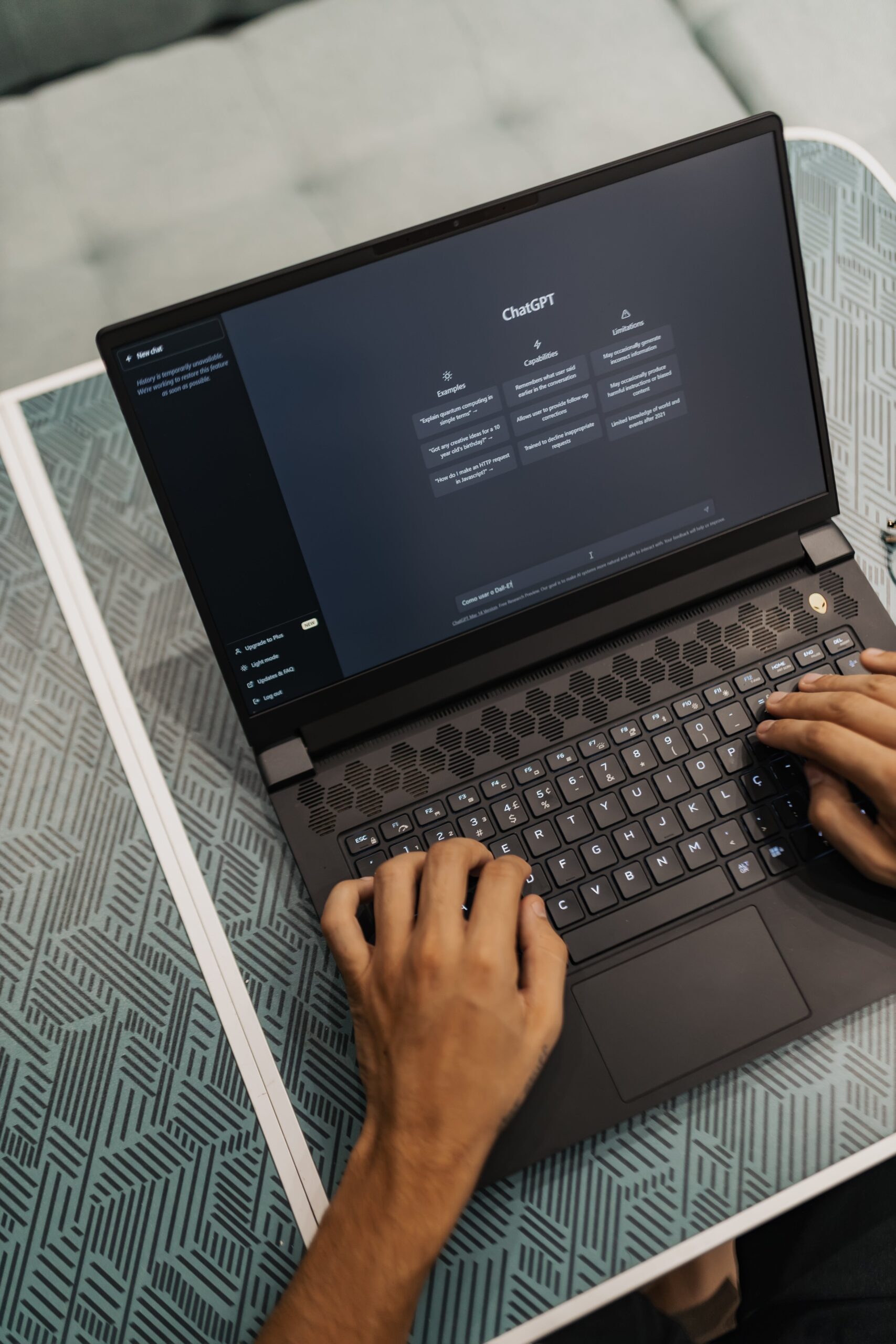
Introduction to ChatGPT Training
Understanding Neural Networks
Evolution of ChatGPT Models (H1)
GPT-1: Foundation of Conversational AI
GPT-2: Advancements in Text Generation
GPT-3: Unprecedented Language Model
- Importance of ChatGPT Training
- Factors Affecting ChatGPT Training Accuracy
- Training Data Quality
- Model Architecture and Hyperparameters
- Best Practices for ChatGPT Training
- Data Preprocessing Techniques
- Hyperparameter Tuning
- Fine-tuning Strategies
- Ethical Considerations in ChatGPT Training
- Future Trends in ChatGPT Training
- Conclusion
- Evolution of ChatGPT Models
- In the realm of Natural Language Processing (NLP), the evolution of ChatGPT models has revolutionized conversational AI. This progression from GPT-1 to GPT-3 has significantly impacted the way machines understand and generate human-like text.
GPT-1: Foundation of Conversational AI
Introduced by OpenAI, GPT-1 laid the groundwork for modern conversational AI. Its architecture was based on a transformer model, enabling it to predict the next word in a sequence, exhibiting rudimentary conversation abilities. Despite its potential, it had limitations in generating coherent and contextually rich responses.
GPT-2: Advancements in Text Generation
GPT-2 marked a leap in text generation capabilities. With 1.5 billion parameters, it demonstrated remarkable improvements in generating coherent and contextually relevant text. This model raised concerns regarding its potential misuse due to the generation of highly convincing fake content.
GPT-3: Unprecedented Language Model
The release of GPT-3 was a watershed moment in NLP. With 175 billion parameters, it showcased astonishing language understanding and generation abilities. GPT-3 displayed the capacity to perform a wide array of language tasks, from translation to code generation, demonstrating its versatility and prowess.
The development of these iterations illustrates the rapid evolution of AI in understanding and producing human-like text, paving the way for diverse applications.
In today’s context, the significance of effective ChatGPT training cannot be overstated. Several factors significantly influence the accuracy and performance of these models.
Factors like the quality of training data, model architecture, and hyperparameter tuning play crucial roles in determining the efficacy of ChatGPT models. Ensuring high-quality and diverse training data, along with optimizing model architecture and hyperparameters, contributes to enhancing the model’s accuracy and robustness.
Adhering to best practices in ChatGPT training is essential for optimal performance. Techniques like data preprocessing, hyperparameter tuning, and fine-tuning strategies refine the model’s capabilities and aid in generating more coherent and contextually relevant responses.
However, the ethical considerations surrounding ChatGPT training warrant attention. Issues related to bias, misinformation, and harmful content generation underscore the importance of responsible AI development and usage.
Looking ahead, the future of ChatGPT training holds promising advancements. Innovations in training methodologies, enhanced ethical frameworks, and the integration of multimodal capabilities are anticipated trends that will shape the landscape of conversational AI.
In conclusion, the evolution of ChatGPT models signifies a remarkable journey in the realm of NLP. Understanding the nuances of training these models, emphasizing ethical considerations, and anticipating future trends are pivotal in harnessing the potential of conversational AI responsibly.
FAQs
Is ChatGPT training similar to traditional machine learning training?
ChatGPT training involves fine-tuning pre-trained language models with specific data, whereas traditional ML training requires building models from scratch using labeled data.
How does data quality impact ChatGPT training?
High-quality, diverse data leads to better model performance, improving the accuracy and relevance of generated text.
What are the ethical concerns associated with ChatGPT models?
Ethical concerns revolve around bias in generated content, misinformation propagation, and potential misuse for malicious purposes.
Can ChatGPT models be fine-tuned for specialized domains?
Yes, ChatGPT models can be fine-tuned using domain-specific data to cater to particular industries or tasks.
What role does hyperparameter tuning play in ChatGPT training?
Optimizing hyperparameters helps in enhancing the model’s performance by fine-tuning its learning process.
Hi There,
I hope you’re well! Your new website venture caught my eye, and I’m genuinely excited about its potential.
As a WordPress expert passionate about creating impactful digital experiences, I see great opportunities to elevate your project.
Could we schedule a quick chat to explore ideas? I’d love to share insights tailored to your goals. Let me know a time that suits you.
Looking forward to the possibility of collaborating!
Best,
Mahmud Ghazni
WordPress Expert Extraordinaire
Email: ghazni@itsyourdev.com
WhatsApp: https://wa.me/8801322311024
وماله ابعتلي لما تبقي فاضي